Modelle
ECOSMO Modelle
ECOSMO II
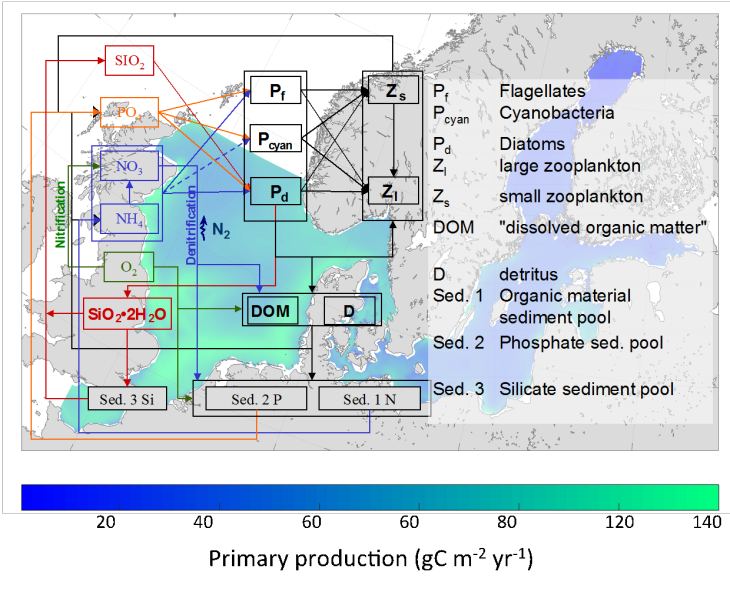
(Graphik: Ute Daewel/ Hereon)
ECOSMO II (ECOSystem Model) ist ein vollständig gekoppeltes physikalisch-biogeochemisches 3D-Modell (Daewel und Schrum, 2013; Schrum et al., 2006a). Das Modell basiert auf dem HAMSOM (HAMburg Shelf Ocean Model) Nord- und Ostseephysik (Schrum, 1997; Schrum und Backhaus, 1999; Barthel et al. 2012). Die biogeochemischen Prozesse in ECOSMO II werden mit 16 Zustandsvariablen simuliert, um die Ökosystemdynamik durch einen funktionalen Gruppenansatz aufzulösen (Abb. 2). Das Modell taxiert zwei funktionelle Zooplanktongruppen, drei Phytoplanktongruppen, den Stickstoff-, Phosphor- und Siliziumkreislauf, Sauerstoff, Detritus, biogenen Opal, gelöste organische Substanz und drei Sedimentgruppen. Das Modell wurde für eine mehrdekadische Langzeitsimulation verwendet und im Detail validiert (Daewel und Schrum, 2013, 2017a). Der validierte 3-d-Modelldatensatz ist über die coastDat-Datenbank in täglicher Auflösung verfügbar (Daewel & Schrum, 2017b), höhere Auflösung auf Anfrage.
ECOSMO E2E
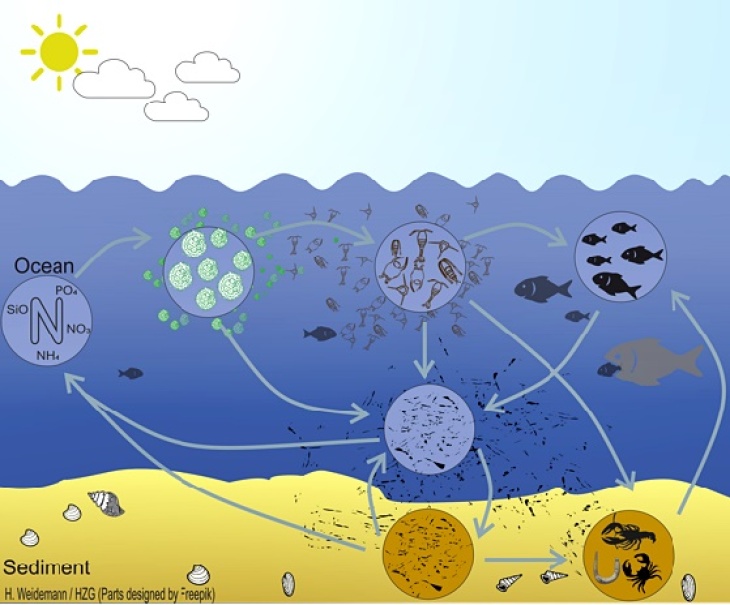
(Graphik: Hendrik Weideman/ Hereon)
Um Fragen zum Nahrungsnetz in der Ostsee zu klären, haben wir das gekoppelte 3D-Ökosystemmodell ECOSMO E2E (Daewel et al. 2019) entwickelt, ein NPZD-Fisch-Modellierungsansatz, der auf dem Ökosystemmodell ECOSMO II (Daewel und Schrum, 2013) basiert. Das Modell stellt sowohl Fische als auch Makrobenthos als funktionale Gruppen dar, die über Räuber-Beute-Beziehungen mit den unteren trophischen Ebenen verbunden sind (Abbildung). Das Modell ermöglicht die Untersuchung von Bottom-up-Auswirkungen auf die Primär- und Sekundärproduktion und die kumulative Dynamik der Fischbiomasse, aber auch von Bottom-up-Mechanismen auf die Produktion der unteren trophischen Ebenen.
ECOSMO-CO2
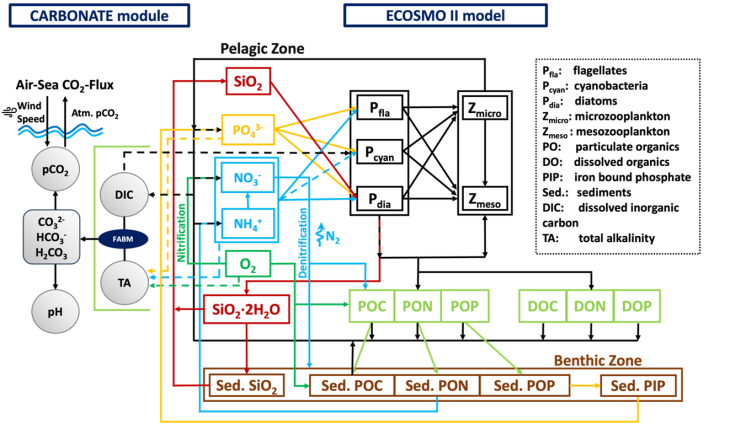
Graphik: Kubilay Timur Demir/ Hereon
ECOSMO-CO2 integriert das Ökosystemmodell ECOSMO II (Daewel und Schrum, 2013) mit einem Kohlenstoffmodul (Blackford und Gilbert, 2007), um Wechselwirkungen zwischen organischem Stoffkreislauf und anorganischer Kohlenstoffchemie zu simulieren. Das Modell verwendet zwei prognostische Tracer – gelösten anorganischen Kohlenstoff (DIC) und Gesamtalkalinität (TA) – um die Karbonatspeziation (CO₂, HCO₃⁻ und CO₃²⁻) und Schlüsselparameter wie den Partialdruck von CO₂ (pCO₂) und den pH-Wert des Meerwassers unter Berücksichtigung der lokalen ozeanographischen Bedingungen zu berechnen. ECOSMO-CO2 wird häufig eingesetzt, um natürliche und anthropogene Einflüsse auf die Kohlenstoffdynamik in Schelfmeeren zu untersuchen. Zu den Anwendungen gehören die Untersuchung der Auswirkungen der Gezeiten auf den CO₂-Austausch (Kossack et al., 2024), die Untersuchung der Rolle der variabler Stöchiometrie in organischen Verbindungen für den CO2 Austausch (Demir et al., 2025) und die Bewertung der Auswirkungen menschlicher Aktivitäten wie der Grundschleppnetzfischerei (Tiwari et al., in Vorbereitung) und ozeanbasierten Technologien zur Kohlendioxidentfernung (CDR) (Liu et al., eingereicht) auf den Kohlenstoffkreislauf des Nordwesteuropäischen Schelfs (NWES).
ECOSMO-ICE
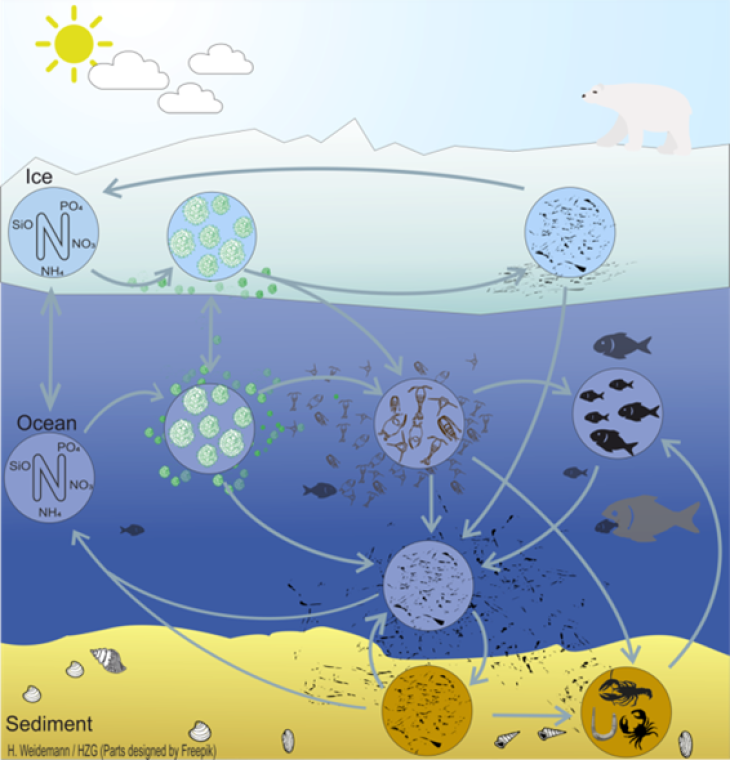
(Graphik: Hendrik Weidemann/ Hereon)
Eines der wichtigsten Merkmale des arktischen Ökosystems ist die Meereisbedeckung und die damit verbundenen mikrobiellen Gemeinschaften, die ein sympagisches Ökosystem bilden. Um ECOSMO E2E (Daewel et al., 2019) auf eisbedeckte Ökosysteme anwendbar zu machen, wurde ein sympagisches Systemmodell entwickelt, das eine Online-Kopplung an das bestehende Modell für die pelagischen und benthischen Systeme ermöglicht. Sechs Zustandsvariablen (Meereisalgen, vier Nährstoffvariablen und eine Detritusgruppe) wurden dem ursprünglichen ECOSMO II-Modell hinzugefügt und Austauschprozesse im Zusammenhang mit Meereisbildung und -schmelze explizit parametrisiert. Eine Anwendung in der Barentssee zeigt, dass das sympagische System das Timing und die Amplitude der pelagischen Primär- und Sekundärproduktion in der Wassersäule beeinflusst. (Benkort et al., 2020; Heath et al., 2022)
ECOSMO-IBM
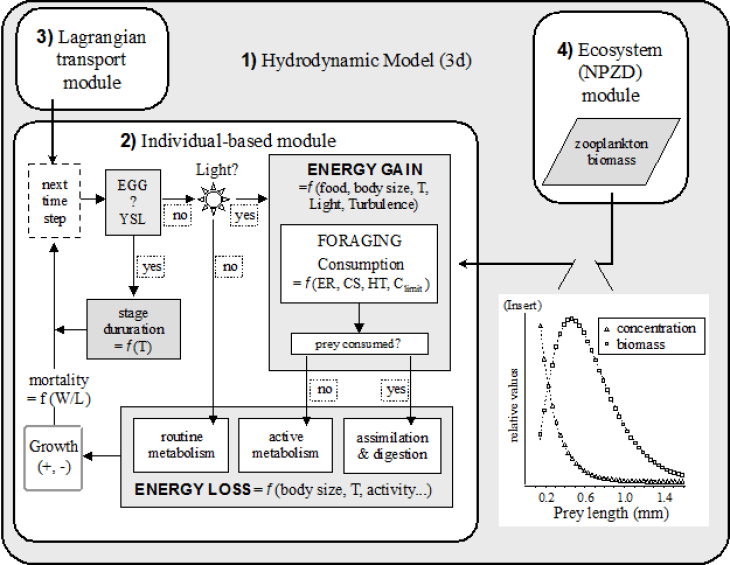
(Graphik: Ute Daewel/ Hereon)
Mit Hilfe räumlich expliziter individuenbasierter Modelle (ECOSMO-IBM) sind wir in der Lage, wissenschaftliche Fragen im Zusammenhang mit frühen Lebensstadien mariner Arten zu beantworten. Mit Hilfe dieses Modells können wir die Flugbahnen einzelner Partikel sowohl in Raum als auch in Zeit verfolgen. Das ECOMSO-IBM-Modell wurde erstmals von Daewel et al. (2008) in einer Anwendung für die Sprotte (Sprattus sprattus) beschrieben. Ein zusätzliches IBM-Submodul für den Kabeljau in der Nordsee wurde von Daewel et al. (2011a) parametrisiert und detailliert beschrieben. Letzteres wurde für eine an die ECOSMO II-Biogeochemie gekoppelte Langzeitsimulation verwendet, um das sich ändernde Überlebenspotenzial der Larven in der Nordsee zu ermitteln (Daewel et al., 2015). Ein statistisches IBM existiert auch für Bachflohkrebse (Daewel et al., 2011b).
Innerhalb des ECOSMO-Rahmens sind weitere Module zum Thema Verbleib und Transport von Schadstoffen verfügbar (z.B. Bieser und Schrum, 2016, 2018 & Bieser et al. 2023). Alle ECOSMO-Module sind mit dem Framework for Aquatic Biogeochemical Models FABM (Bruggeman und Bolding, 2014) gekoppelt.
Referenzen
- Bruggeman, J., and Bolding, K. 2014. A general framework for aquatic biogeochemical models. Environmental Modelling and Software, 61: 249–265. Elsevier Ltd. http://dx.doi.org/10.1016/j.envsoft.2014.04.002
- Blackford, J. C., & Gilbert, F. J. (2007). pH variability and CO2 induced acidification in the North Sea. Journal of Marine Systems, 64(1-4), 229-241
- Barthel, K., Daewel, U., Pushpadas, D., Schrum, C.Årthun, M., & Wehde, H. (2012): Resolving frontal structures: On the computational costs and pay-off using a less diffusive but computational more expensive advection scheme. Ocean Dynamics, doi:10.1007/s10236-012-0578-9
- Benkort, D., Daewel, U., Heath, M., and Schrum, C. 2020. On the Role of Biogeochemical Coupling Between Sympagic and Pelagic Ecosystem Compartments for Primary and Secondary Production in the Barents Sea. https://www.frontiersin.org/article/10.3389/fenvs.2020.548013
- Bieser, J., Amptmeijer, D.J., Daewel, U., Kuss, J., Soerensen, A.L., & Schrum, C. (2023): The 3D biogeochemical marine mercury cycling model MERCY v2.0 – linking atmospheric Hg to methylmercury in fish. Geosci. Model Dev., 16, 2649–2688, doi:10.5194/gmd-16-2649-2023
- Daewel, U., Peck, M.A., Kühn, W., St. John, M.A., Alekseeva, I., & Schrum, C. (2008): Coupling ecosystem and individual-based models to simulate the influence of environmental variability on potential growth and survival of larval sprat ( Sprattus sprattus L.) in the North Sea. Fish. Oceanogr. 17, 333–351, doi:10.1111/j.1365-2419.2008.00482.x
- Daewel, U., Peck, M.A.M.A., & Schrum, C. (2011): Life history strategy and impacts of environmental variability on early life stages of two marine fishes in the North Sea: an individual-based modelling approach. Can. J. Fish. Aquat. Sci. 68, 426–443, doi:10.1139/F10-164
- Daewel, U., & Schrum, C.(2013): Simulating long-term dynamics of the coupled North Sea and Baltic Sea ecosystem with ECOSMO II: Model description and validation. Journal of Marine Systems 119, 30–49, doi:10.1016/j.jmarsys.2013.03.008
- Daewel, U., & Schrum, C. (2017): Low-frequency variability in North Sea and Baltic Sea identified through simulations with the 3-D coupled physical-biogeochemical model ECOSMO. Earth Syst. Dyn. 8, doi:10.5194/esd-8-801-2017
- Daewel, U., Schrum, C., and MacDonald, J. I. 2019. Towards end-to-end (E2E) modelling in a consistent NPZD-F modelling framework (ECOSMO E2E-v1.0): Application to the North Sea and Baltic Sea. Geoscientific Model Development, 12: 1765–1789
- Daewel, U., Schrum, C., & Gupta, A.K. (2015): The predictive potential of early life stage individual-based models (IBMs): an example for Atlantic cod Gadus morhua in the North Sea. Mar. Ecol. Prog. Ser. 534, 199–219, doi:10.3354/meps11367
- Daewel, U., Schrum, C., & Temming, A. (2011): Towards a more complete understanding of the life cycle of brown shrimp (Crangon crangon): Modelling passive larvae and juvenile transport in combination with physically forced vertical juvenile migration. Fish. Oceanogr. 20, doi:10.1111/j.1365-2419.2011.00597.x
- Demir, K. T., Mathis, M., Kossack, J., Liu, F., Daewel, U., Stegert, C., Thomas, H., & Schrum, C. (2024). Variable organic matter stoichiometry enhances the biological drawdown of CO2 in the Northwest European shelf seas. EGUsphere, 2024, 1-47
- Heath, M. R., Benkort, D., Brierley, A. S., Daewel, U., Laverick, J. H., Proud, R., and Speirs, D. C. 2022. Ecosystem approach to harvesting in the Arctic: Walking the tightrope between exploitation and conservation in the Barents Sea. Ambio, 51: 456–470. https://doi.org/10.1007/s13280-021-01616-9
- Kossack, J., Mathis, M., Daewel, U., Liu, F., Demir, K. T., Thomas, H., & Schrum, C. (2024). Tidal impacts on air-sea CO2 exchange on the North-West European shelf. Frontiers in Marine Science, 11, 1406896
- Liu, F., Daewel, U., Kossack, J., Demir, K. T., Thomas, H., & Schrum, C. (2024). Evaluating ocean alkalinity enhancement as a carbon dioxide removal strategy in the North Sea. (Submitted to Biogeosciences)
- Peck, M.A., & Daewel, U. (2007): Physiologically based limits to food consumption, and individual-based modeling of foraging and growth of larval fishes. Mar. Ecol. Prog. Ser. 347, 171–183, doi:10.3354/meps06976
- Schrum, C. (1997): A coupled ice-ocean model for the North Sea and the Baltic Sea. Sensitivity of North Sea, Baltic Sea and Black Sea to anthropogenic and climatic changes. In: Özsoy, E., & Mukaelyan, A. (Eds.): Sensitivity of North Sea, Baltic Sea and Black Sea to Anthropogenic and Climatic Changes. Nato ASI Series. Kluwer Academic Publishers, Dordrecht, pp. 311–325.
- Schrum, C. (2001): Regionalization of climate change for the North Sea and Baltic Sea. Clim. Res. 18, 31–37.
- Schrum, C., Alekseeva, I., & St. John, M. (2006): Development of a coupled physical–biological ecosystem model ECOSMO. J. Mar. Syst. 61, 79–99, doi:10.1016/j.jmarsys.2006.01.005
- Schrum, C., & Backhaus, J.O. (1999): Sensitivity of atmosphere-ocean heat exchange and heat content in North Sea and Baltic Sea. A comparitive assessment. Tellus 51A. 526-549.
- Tiwari, P., Porz, L., Daewel, U., Kossack, J., Liu, F., Demir, K. T., Zhang, W., & Schrum, C.. Constraining Bottom Trawling Effects on North Sea Productivity: A Modeling Approach. (in preparation).
- Daewel, U., & Schrum, C. (2017): ECOSMO II hindcast simulations for the North Sea and Baltic Sea (1948-2008). World Data Center for Climate (WDCC) at DKRZ. doi:10.1594/WDCC/ECOSMOII_NCEP.1948-2008
- Samuelsen, A., Schrum, C., Castano Primo, R., Yumruktepe, C.V., & Daewel, U. (2018): A model hindcast of bottom environmental conditions in the North Atlantic Ocean 1948 to 2014. Nansen Environmental and Remote Sensing Center, PANGAEA, doi:10.1594/PANGAEA.889396
- Alekseeva, I., Jarsjö, J., Destouni, G., & Schrum, C. (2009): Reproducing the Aral Sea water budget and sea–groundwater dynamics between 1979 and 1993 using a coupled 3-D sea-ice– groundwater model. doi:10.1016/j.jmarsys.2008.03.018
- Alekseeva, I., & Schrum, C. (2008): Historical reconstruction of the Aral Sea shrinking by a full 3-d wetting and drying model ECOSMO. Geosci. Model Dev. Discuss., 1, S92–S98
- Bieser, J., & Schrum, C. (2016): Impact of Marine Mercury Cycling on Coastal Atmospheric Mercury Concentrations in the North- and Baltic Sea region. Elementa, Science of the Anthropocene, 4:000111, doi:10.12952/journal.elementa.000111
- Bieser, J., & Schrum, C. (2018): Evaluation of the Impact of Air-Sea Exchange on Atmospheric Mercury Concentrations. In: Mensink C., & Kallos G. (eds): Air Pollution Modeling and its Application XXV. ITM 2016. Springer Proceedings in Complexity. Springer, Cham, doi:10.1007/978-3-319-57645-9_69
- Burt, W.J., Thomas, H., Pätsch, J., Omar, A.M., Schrum, C., Daewel, U., Brenner, H., & Baar, de H.J.W. (2014): Radium isotopes as a tracer of sediment-water column exchange in the North Sea. Global Biogeochem. Cycles, 28, doi:10.1002/2014GB004825
- Chust, G., Allen, J.I., Bopp, L., Schrum, C., Holt, J., Tsiaras, K., Zavatarelli, M., Chifflet, M., Cannaby, H., Dadou, I., Daewel, U., Wakelin, S.L., Machu, E., Pushpadas, D., Butenschon, M., Artioli, Y., Petihakis, G., Smith, C., Garçon, V., Goubanova, K., Le Vu, B., Fach, B.A., Salihoglu, B., Clementi, E., & Irigoien, X. (2014): Biomass changes and trophic amplification of plankton in a warmer ocean. Glob. Chang. Biol. 20, 2124–39, doi:10.1111/gcb.12562
- Christensen, A., Daewel, U., Jensen, H., Mosegaard, H., St. John, M., & Schrum, C. (2007): Hydrodynamic backtracking of fish larvae by individual-based modelling. Mar. Ecol. Prog. Ser., 347, 221-232
- Christensen, A., Jensen, H., Mosegaard, H., St. John, M., & Schrum, C. (2008): Sandeel larval transport patterns in North Sea from an individual-based hydrodynamic egg and larval model. Canadian Journal of Fisheries and Aquatic Sciences. 65-7, 1498-1511
- Daewel, U., Peck, M.A., Schrum, C., & St. John, M. (2008): How best to include the effects of climate-driven forcing on prey fields in larval fish individual based models. Journal of Plankton Research, 30, 1-5
- Harms, I., Schrum, C., & Hatten, K. (2005): Numerical sensitivity studies on the variability of climate relevant processes in the Barents Sea. JGR, J. Geophys. Res., 110, C06002, doi:10.1029/2004JC002559
- Holt, J., Schrum, C., Cannaby, H., Daewel, U., Allen, I., Artioli, Y., Bopp, L., Butenschon, M., Fach, B.A., Harle, J., Pushpadas, D., Salihoglu, B., & Wakelin, S. (2016): Potential impacts of climate change on the primary production of regional seas: A comparative analysis of five European seas. Prog. Oceanogr. 140, doi:10.1016/j.pocean.2015.11.004
- Holt, J., Schrum, C., Cannaby, H., Daewel, U., Allen, I., Artioli, Y., Bopp, L., Butenschon, M., Fach, B., Harle, J., Pushpadas, D., Salihoglu, B., & Wakelin, S. (2014): Physical processes mediating climate change impacts on regional sea ecosystems, Biogeosciences Discuss., 11, 1909-1975, www.biogeosciences-discuss.net/11/1909/2014/, doi:10.5194/bgd-11-1909-2014
- Hufnagl, M., Payne, M., Lacroix, G., Bolle, L.J., Daewel, U., Dickey-Collas, M., Gerkema, T., Huret, M., Janssen, F., Kreus, M., Pätsch, J., Pohlmann, T., Ruardij, P., Schrum, C., Skogen, M.D., Tiessen, M.C.H., Petitgas, P., Beek, van J.K.L., Veer, van der H.W., & Callies, U. (2017): Variation that can be expected when using particle tracking models in connectivity studies. J. Sea Res., doi:10.1016/j.seares.2017.04.009
- Jansen, T., Kristensen, K., Payne, M., Edwards, M., Schrum, C., & Pitois, S. (2012): Long-term Retrospective Analysis of Mackerel Spawning in the North Sea: A New Time Series and Modeling Approach to CPR Data. PLoSOne, Marine and Aquatic Sciences, 7, doi:10.1371/journal.pone.0038758
- Janssen, F., Schrum, C., Hübner, U., & Backhaus, J.O. (2001): Uncertainty analysis of a decadal simulation with a regional ocean model for the North Sea and Baltic Sea. Clim. Res. 18, 55–62
- Jarsjö, J., Alekseeva, I., Schrum, C., & Destouni, G. (2005): Simulation of groundwater - sea water interactions in the Aral Sea basin by a coupled water balance model. Calibration and Reliability in Groundwater Modelling: From Uncertainty to Decision Making (Proceedings of ModelCARE’2005, The Hague, The Netherlands, June 2005). IAHS Publ. 304, pp.261–267
- Maar, M., Butenschön, M., Daewel, U., Eggert, A., Fan, W., Hjøllo, S.S., Hufnagl, M., Huret, M., Ji, R., Lacroix, G., Peck, M.A., Radtke, H., Sailley, S., Sinerchia, M., Skogen, M.D., Travers-Trolet, M., Troost, T.A., & Wolfshaar, van de K. (2018): Responses of summer phytoplankton biomass to changes in top-down forcing: Insights from comparative modelling. Ecol. Modell. 376, 54–67, doi:10.1016/j.ecolmodel.2018.03.003
- Pushpadas, D., Schrum, C., & Daewel, U. (2015): Projected climate change impacts on North Sea and Baltic Sea: CMIP3 and CMIP5 model based scenarios. Biogeoscience discussions, 12, 12229-12279, 2015 www.biogeosciences-discuss.net/12/12229/2015/, doi:10.5194/bgd-12-12229-2015
- Rindorf, A., Jensen, H., & Schrum, C. (2008): Growth, temperature and density relationships of North Sea. Canadian Journal of Fisheries Res, 456-470
- Schrum, C., Siegismund, F., & St. John, M. (2003): Decadal Variations in the stratification and circulation patterns of the North Sea. Are the 90’s unusual? ICES Symposium of Hydrobiological Variability in the ICES area 1990-1999, ICES Journal of Marine Science, Symposia series, Vol. 219, 121-131
- Schrum, C., St. John, M., & Alekseeva, I. (2006): ECOSMO, a coupled ecosystem model of the North Sea and Baltic Sea: Part II. Spatial-seasonal characteristics in the North Sea as revealed by EOF analysis. Journal of Marine Systems, doi:10.1016/j.jmarsys.2006.01.004
- Schrum, C., Harms, I., & Hatten, K. (2005): Modelling Air-sea exchange in the Barents Sea By using a coupled regional ice-ocean model. Meteorologische Zeitschrift, 14, No. 6, 1-3, doi:10.1127/0941-2948/2005/0075
- Skogen, M.D., Drinkwater, K., Hjøllo, S.S., & Schrum, C. (2011): North Sea sensitivity to atmospheric forcing. Journal of Marine Systems, doi:10.1016/j.jmarsys.2010.12.008
- Årthun, M., Bellerby, R.G.J., Omar, A.M., & Schrum, C. (2012): Air-sea CO2 fluxes in the Barents Sea as determined from empirical relationships. Journal of Marine Systems, doi:10.1016/j.jmarsys.2012.03.005
- Årthun, M., Ingvaldsen, R., Smedsrud, L.H., & Schrum, C. (2011): Dense Water formation and circulation in the Barents Sea. Deep Sea Research I 58, 801-817
- Årthun, M., & Schrum, C. (2010): Ocean surface heat flux variability in the Barents Sea. Journal of Marine Systems, 83, 88-98